Update on: July 18, 2024
Integrated analyses highlight interactions between the 3D genome and DNA, RNA and epigenomic alterations
The impact of variations in the three-dimensional structure of the genome has been recognized, but solid cancer tissue studies are limited.
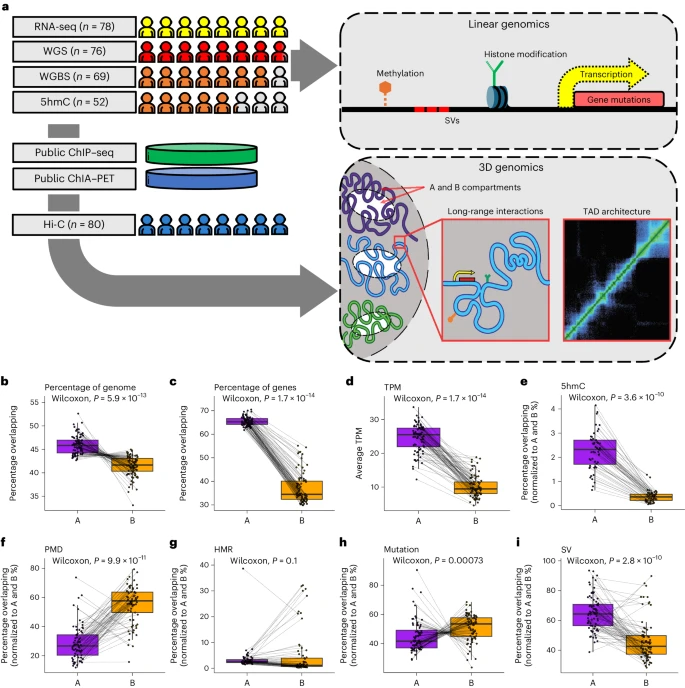
Here, we performed integrated deep Hi-C sequencing with matched whole-genome sequencing, whole-genome bisulfite sequencing, 5-hydroxymethylcytosine (5hmC) sequencing and RNA sequencing across a cohort of 80 biopsy samples from patients with metastatic castration-resistant prostate cancer. Dramatic differences were present in gene expression, 5-methylcytosine/5hmC methylation and in structural variation versus mutation rate between A and B (open and closed) chromatin compartments. A subset of tumors exhibited depleted regional chromatin contacts at the AR locus, linked to extrachromosomal circular DNA (ecDNA) and worse response to AR signaling inhibitors.
We also identified topological subtypes associated with stark differences in methylation structure, gene expression and prognosis. Our data suggested that DNA interactions may predispose to structural variant formation, exemplified by the recurrent TMPRSS2–ERG fusion. This comprehensive integrated sequencing effort represents a unique clinical tumor resource. Reference
ezSingleCell: an integrated one-stop single-cell and spatial omics analysis platform for bench scientists
ezSingleCell is an interactive and easy-to-use application for analysing various single-cell and spatial omics data types without requiring prior programing knowledge.
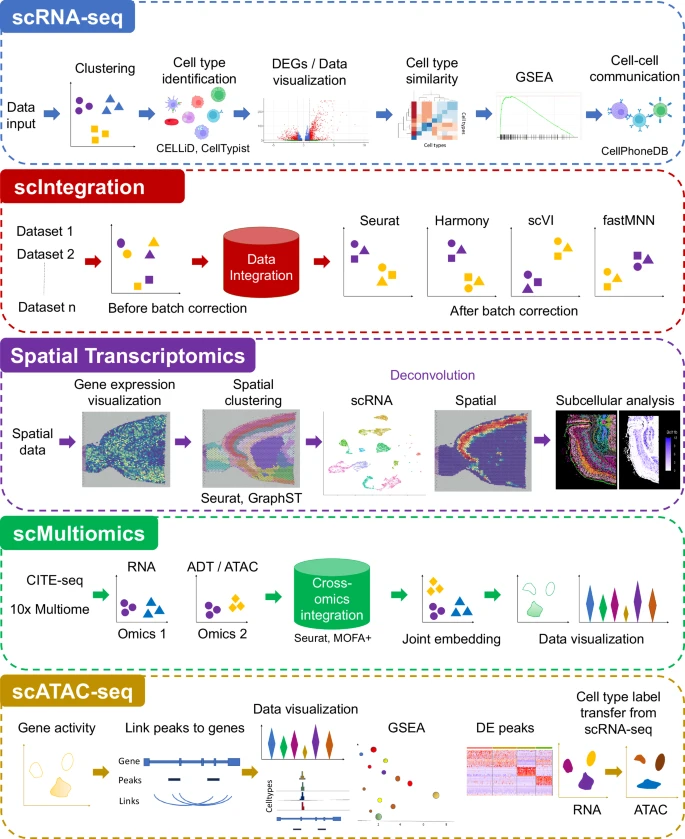
It combines the best-performing publicly available methods for in-depth data analysis, integration, and interactive data visualization. ezSingleCell consists of five modules, each designed to be a comprehensive workflow for one data type or task. In addition, ezSingleCell allows crosstalk between different modules within a unified interface.
Acceptable input data can be in a variety of formats while the output consists of publication ready figures and tables. In-depth manuals and video tutorials are available to guide users on the analysis workflows and parameter adjustments to suit their study aims. ezSingleCell’s streamlined interface can analyse a standard scRNA-seq dataset of 3000 cells in less than five minutes. Reference
Multi-modal generative modeling for joint analysis of single-cell T cell receptor and gene expression data
Recent advances in single-cell immune profiling have enabled the simultaneous measurement of transcriptome and T cell receptor (TCR) sequences, offering great potential for studying immune responses at the cellular level.
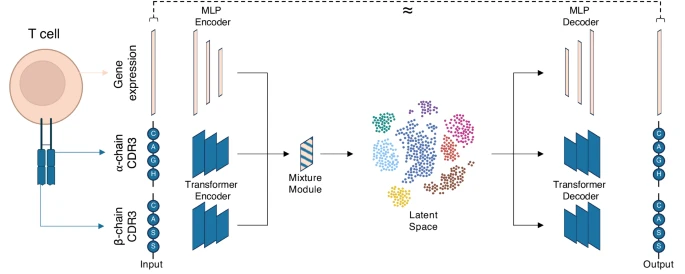
However, integrating these diverse modalities across datasets is challenging due to their unique data characteristics and technical variations. Here, to address this, we develop the multimodal generative model mvTCR to fuse modality-specific information across transcriptome and TCR into a shared representation. Our analysis demonstrates the added value of multimodal over unimodal approaches to capture antigen specificity.
Notably, we use mvTCR to distinguish T cell subpopulations binding to SARS-CoV-2 antigens from bystander cells. Furthermore, when combined with reference mapping approaches, mvTCR can map newly generated datasets to extensive T cell references, facilitating knowledge transfer. In summary, we envision mvTCR to enable a scalable analysis of multimodal immune profiling data and advance our understanding of immune responses. Reference
Multi-omics signatures reveal genomic and functional heterogeneity of Cutibacterium acnes in normal and diseased skin
Cutibacterium acnes is the most abundant bacterium of the human skin microbiome since adolescence, participating in both skin homeostasis and diseases.

Here, we demonstrate individual and niche heterogeneity of C. acnes from 1,234 isolate genomes. Skin disease (atopic dermatitis and acne) and body site shape genomic differences of C. acnes, stemming from horizontal gene transfer and selection pressure. C. acnes harbors characteristic metabolic functions, fewer antibiotic resistance genes and virulence factors, and a more stable genome compared with Staphylococcus epidermidis. Integrated genome, transcriptome, and metabolome analysis at the strain level unveils the functional characteristics of C. acnes.
Consistent with the transcriptome signature, C. acnes in a sebum-rich environment induces toxic and pro-inflammatory effects on keratinocytes. L-carnosine, an anti-oxidative stress metabolite, is up-regulated in the C. acnes metabolome from atopic dermatitis and attenuates skin inflammation. Collectively, our study reveals the joint impact of genes and the microenvironment on C. acnes function. Reference
A metabolomics pipeline highlights microbial metabolism in bloodstream infections
The growth of antimicrobial resistance (AMR) highlights an urgent need to identify bacterial pathogenic functions that may be targets for clinical intervention. Although severe infections profoundly alter host metabolism, prior studies have largely ignored microbial metabolism in this context.

Here, we describe an iterative, comparative metabolomics pipeline to uncover microbial metabolic features in the complex setting of a host and apply it to investigate gram-negative bloodstream infection (BSI) in patients. We find elevated levels of bacterially derived acetylated polyamines during BSI and discover the enzyme responsible for their production (SpeG).
Blocking SpeG activity reduces bacterial proliferation and slows pathogenesis. Reduction of SpeG activity also enhances bacterial membrane permeability and increases intracellular antibiotic accumulation, allowing us to overcome AMR in culture and in vivo. This study highlights how tools to study pathogen metabolism in the natural context of infection can reveal and prioritize therapeutic strategies for addressing challenging infections. Reference
TMO-Net: an explainable pretrained multi-omics model for multi-task learning in oncology
Cancer is a complex disease composing systemic alterations in multiple scales.
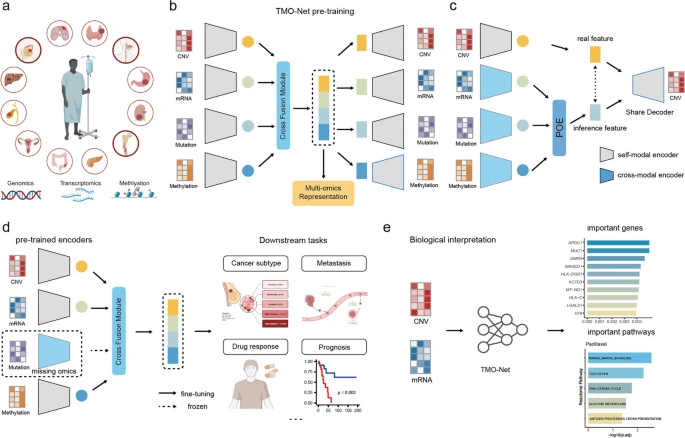
In this study, we develop the Tumor Multi-Omics pre-trained Network (TMO-Net) that integrates multi-omics pan-cancer datasets for model pre-training, facilitating cross-omics interactions and enabling joint representation learning and incomplete omics inference. This model enhances multi-omics sample representation and empowers various downstream oncology tasks with incomplete multi-omics datasets.
By employing interpretable learning, we characterize the contributions of distinct omics features to clinical outcomes. The TMO-Net model serves as a versatile framework for cross-modal multi-omics learning in oncology, paving the way for tumor omics-specific foundation models. Reference
SIMS: A deep-learning label transfer tool for single-cell RNA sequencing analysis
Cell atlases serve as vital references for automating cell labeling in new samples, yet existing classification algorithms struggle with accuracy.

Here we introduce SIMS (scalable, interpretable machine learning for single cell), a low-code data-efficient pipeline for single-cell RNA classification. We benchmark SIMS against datasets from different tissues and species. We demonstrate SIMS’s efficacy in classifying cells in the brain, achieving high accuracy even with small training sets (<3,500 cells) and across different samples. SIMS accurately predicts neuronal subtypes in the developing brain, shedding light on genetic changes during neuronal differentiation and postmitotic fate refinement.
Finally, we apply SIMS to single-cell RNA datasets of cortical organoids to predict cell identities and uncover genetic variations between cell lines. SIMS identifies cell-line differences and misannotated cell lineages in human cortical organoids derived from different pluripotent stem cell lines. Altogether, we show that SIMS is a versatile and robust tool for cell-type classification from single-cell datasets. Reference
Cell-type-specific consequences of mosaic structural variants in hematopoietic stem and progenitor cells
The functional impact and cellular context of mosaic structural variants (mSVs) in normal tissues is understudied. Utilizing Strand-seq,
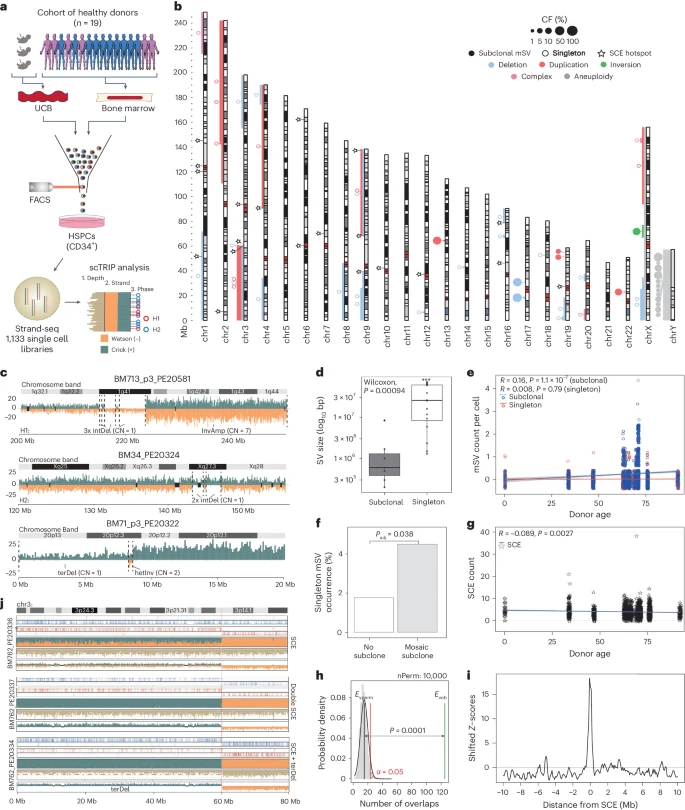
we sequenced 1,133 single-cell genomes from 19 human donors of increasing age, and discovered the heterogeneous mSV landscapes of hematopoietic stem and progenitor cells. While mSVs are continuously acquired throughout life, expanded subclones in our cohort are confined to individuals >60. Cells already harboring mSVs are more likely to acquire additional somatic structural variants, including megabase-scale segmental aneuploidies. Capitalizing on comprehensive single-cell micrococcal nuclease digestion with sequencing reference data, we conducted high-resolution cell-typing for eight hematopoietic stem and progenitor cells.
Clonally expanded mSVs disrupt normal cellular function by dysregulating diverse cellular pathways, and enriching for myeloid progenitors. Our findings underscore the contribution of mSVs to the cellular and molecular phenotypes associated with the aging hematopoietic system, and establish a foundation for deciphering the molecular links between mSVs, aging and disease susceptibility in normal tissues. Reference
A genomic compendium of cultivated human gut fungi characterizes the gut mycobiome and its relevance to common diseases
The gut fungal community represents an essential element of human health, yet its functional and metabolic potential remains insufficiently elucidated, largely due to the limited availability of reference genomes.

To address this gap, we presented the cultivated gut fungi (CGF) catalog, encompassing 760 fungal genomes derived from the feces of healthy individuals. This catalog comprises 206 species spanning 48 families, including 69 species previously unidentified. We explored the functional and metabolic attributes of the CGF species and utilized this catalog to construct a phylogenetic representation of the gut mycobiome by analyzing over 11,000 fecal metagenomes from Chinese and non-Chinese populations.
Moreover, we identified significant common disease-related variations in gut mycobiome composition and corroborated the associations between fungal signatures and inflammatory bowel disease (IBD) through animal experimentation. These resources and findings substantially enrich our understanding of the biological diversity and disease relevance of the human gut mycobiome. Reference
Genome-wide analysis in over 1 million individuals of European ancestry yields improved polygenic risk scores for blood pressure traits
Hypertension affects more than one billion people worldwide. Here we identify 113 novel loci, reporting a total of 2,103 independent genetic signals (P < 5 × 10−8) from the largest single-stage blood pressure (BP) genome-wide association study to date (n = 1,028,980 European individuals).
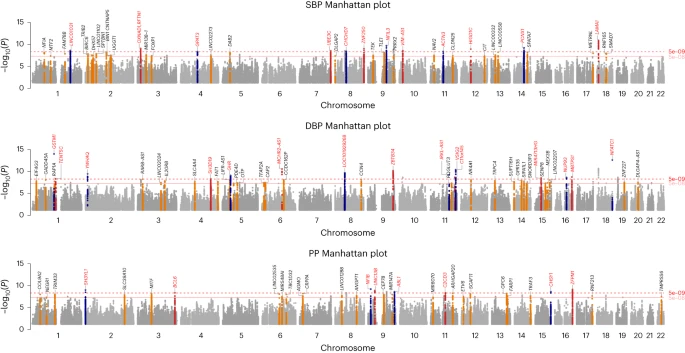
These associations explain more than 60% of single nucleotide polymorphism-based BP heritability. Comparing top versus bottom deciles of polygenic risk scores (PRSs) reveals clinically meaningful differences in BP (16.9 mmHg systolic BP, 95% CI, 15.5–18.2 mmHg, P = 2.22 × 10−126) and more than a sevenfold higher odds of hypertension risk (odds ratio, 7.33; 95% CI, 5.54–9.70; P = 4.13 × 10−44) in an independent dataset.
Adding PRS into hypertension-prediction models increased the area under the receiver operating characteristic curve (AUROC) from 0.791 (95% CI, 0.781–0.801) to 0.826 (95% CI, 0.817–0.836, ∆AUROC, 0.035, P = 1.98 × 10−34). We compare the 2,103 loci results in non-European ancestries and show significant PRS associations in a large African-American sample. Secondary analyses implicate 500 genes previously unreported for BP. Our study highlights the role of increasingly large genomic studies for precision health research. Reference
hadge: a comprehensive pipeline for donor deconvolution in single-cell studies
Single-cell multiplexing techniques (cell hashing and genetic multiplexing) combine multiple samples, optimizing sample processing and reducing costs.
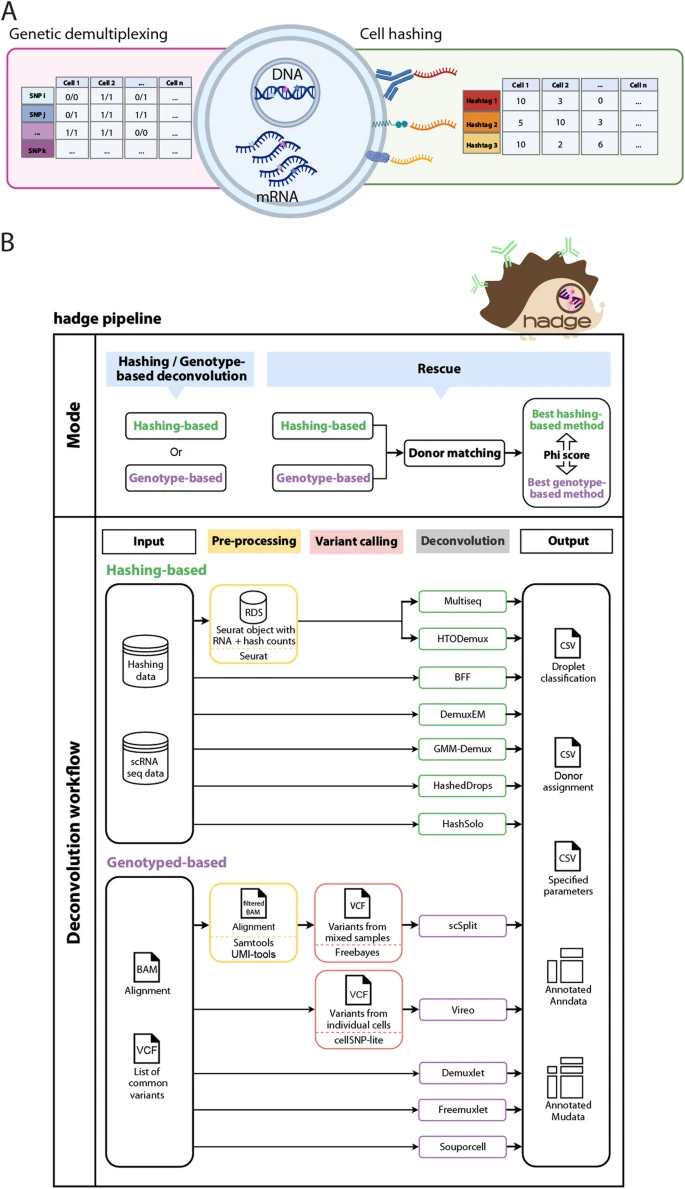
Cell hashing conjugates antibody-tags or chemical-oligonucleotides to cell membranes, while genetic multiplexing allows to mix genetically diverse samples and relies on aggregation of RNA reads at known genomic coordinates.
We develop hadge (hashing deconvolution combined with genotype information), a Nextflow pipeline that combines 12 methods to perform both hashing- and genotype-based deconvolution. We propose a joint deconvolution strategy combining best-performing methods and demonstrate how this approach leads to the recovery of previously discarded cells in a nuclei hashing of fresh-frozen brain tissue. Reference
GWAS identify 95 risk loci and provide insights into the neurobiology of post-traumatic stress disorder
Post-traumatic stress disorder (PTSD) genetics are characterized by lower discoverability than most other psychiatric disorders. The contribution to biological understanding from previous genetic studies has thus been limited.
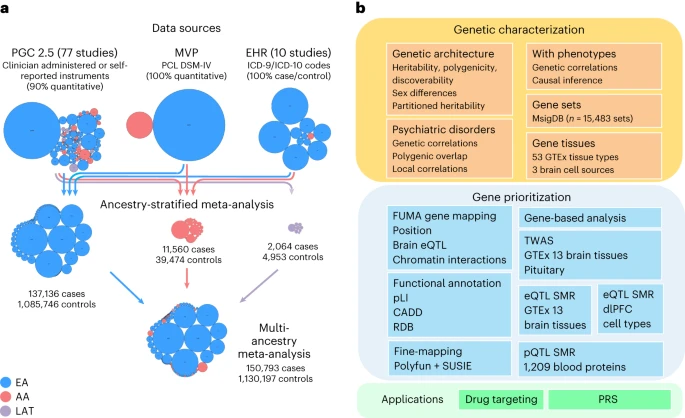
We performed a multi-ancestry meta-analysis of genome-wide association studies across 1,222,882 individuals of European ancestry (137,136 cases) and 58,051 admixed individuals with African and Native American ancestry (13,624 cases). We identified 95 genome-wide significant loci (80 new).
Convergent multi-omic approaches identified 43 potential causal genes, broadly classified as neurotransmitter and ion channel synaptic modulators (for example, GRIA1, GRM8 and CACNA1E), developmental, axon guidance and transcription factors (for example, FOXP2, EFNA5 and DCC), synaptic structure and function genes (for example, PCLO, NCAM1 and PDE4B) and endocrine or immune regulators (for example, ESR1, TRAF3 and TANK). Additional top genes influence stress, immune, fear and threat-related processes, previously hypothesized to underlie PTSD neurobiology. These findings strengthen our understanding of neurobiological systems relevant to PTSD pathophysiology, while also opening new areas for investigation. Reference
A pan-cancer analysis of the microbiome in metastatic cancer
Microbial communities are resident to multiple niches of the human body and are important modulators of the host immune system and responses to anticancer therapies.

Recent studies have shown that complex microbial communities are present within primary tumors. To investigate the presence and relevance of the microbiome in metastases, we integrated mapping and assembly-based metagenomics, genomics, transcriptomics, and clinical data of 4,160 metastatic tumor biopsies. We identified organ-specific tropisms of microbes, enrichments of anaerobic bacteria in hypoxic tumors, associations between microbial diversity and tumor-infiltrating neutrophils, and the association of Fusobacterium with resistance to immune checkpoint blockade (ICB) in lung cancer.
Furthermore, longitudinal tumor sampling revealed temporal evolution of the microbial communities and identified bacteria depleted upon ICB. Together, we generated a pan-cancer resource of the metastatic tumor microbiome that may contribute to advancing treatment strategies. Reference